How Lorenzo Bomba is using rare genetic variants to understand blood metabolites
Lorenzo Bomba was a postdoctoral fellow in the Soranzo group, which uses large-scale genomic analysis to study the human variation linked to cardiometabolic traits and diseases. He recently published the results of an Open Targets project which found new associations between genes and blood metabolites in the American Journal of Human Genetics.
To find out more, I sat down with him to chat about the project and its implications for target identification and drug discovery.
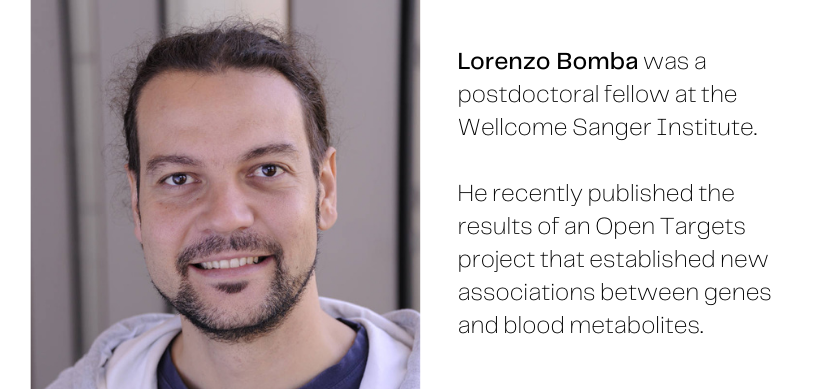
What was this study about?
We looked at associations between rare genetic variants and metabolite levels in a healthy group of individuals. Metabolites are substances that are produced during metabolism. For example, lactic acid is a metabolite that is formed when you exercise.
More specifically, in this study we were looking at blood metabolites from participants in the INTERVAL study. Since the samples can be collected very easily from blood donations, we were able to study almost 1,000 metabolites in over 6,700 individuals — this is one of the largest sequencing metabolomics studies to date. Another advantage of the INTERVAL study is that we were able to study both metabolite as well as protein levels (using the SOMAlogic platform).
Why did you choose to focus on rare variants?
Most studies of this kind have focused on common genetic variation; we chose to focus on rare genetic variants, particularly those that cause a loss of function of the gene, because these are particularly interesting for drug discovery. Rare variants include those that completely inactivate genes that might affect metabolite levels; this could be viewed as a natural experiment that could show what would happen if we used a drug to interfere in the process. A good example are variants in the PCSK9 and ANGPTL3 genes.
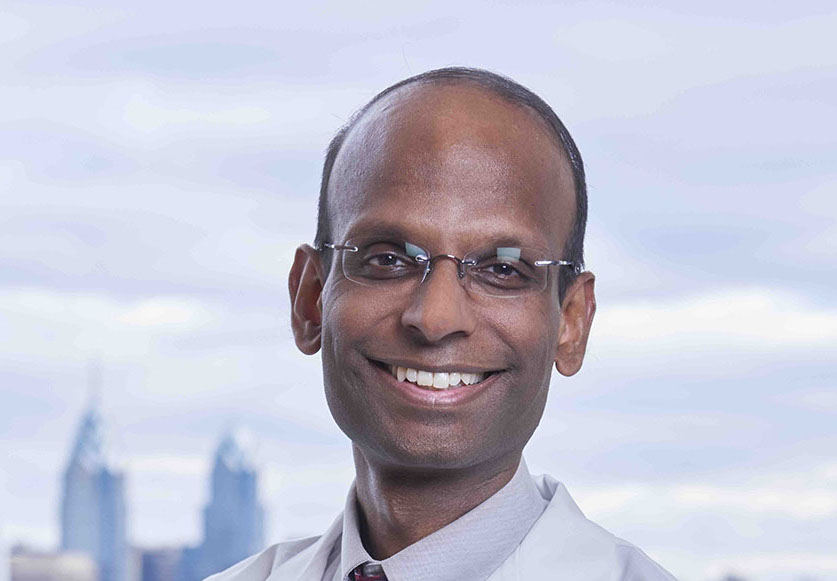
Why study metabolites?
Studying metabolites allows us to dig into the biological processes that underlie disease. Metabolite levels indicate gene activity in metabolism, and can therefore provide information about how a certain gene might be contributing to a disease or phenotype. Metabolite levels can also improve our understanding of how diseases are related, for example by helping to define a spectrum from rare to common diseases.
This is critical information for drug discovery: studying metabolites will suggest new drug targets, and will improve our knowledge of disease biology.
What was innovative about your study?
Rare variants are so-called because each individual mutation occurs infrequently, which makes it difficult to perform statistical analyses. However, by pooling rare variants, we have more statistical power to detect which ones are associated with a particular phenotype.
The difficulty is to decide which variants to pool for analysis, and then to unpick which ones were most contributing to a positive association signal. We developed a novel method to do this.
First, we aggregated the variants using exons as a test unit and we set the threshold to 30 rare variants per unit to limit the noise. We also aggregated variants across the entire gene. The former approach allows us to identify associations when contributing variants are clustering within a particular domain, while the latter helps when contributing variants are spread across the gene.
This combined approach, the selection of different types of predicted functional variants and multiple statistical models, allowed us to explore a variety of allelic architectures and to discover a number of associations between metabolites and test units.
Finally, we created an algorithm to identify the variants that were more likely to contribute to the associations, termed “driver variants”.
This is one of the largest sequencing metabolomics studies to date.
What did you find?
We identified novel associations between variants and metabolites, which provided evidence for 40 gene-metabolite associations, between 27 genes and 38 metabolites. Of these, 16 gene-metabolite associations were completely novel. We also replicated 28 of these associations using a non-overlapping sample of participants with WGS data from the INTERVAL study.
We searched the scientific literature to gather information about these associations, and we found a lot of corroborating evidence. Using the Open Targets Platform, we found that most associations had a clear biochemical justification why alterations would affect metabolic reactions. In fact, 66% of the associations implicated gene targets of approved drugs or drug-like compounds. This is a good indication that our method is working as expected by identifying biologically relevant targets.
Browsing associations of rare variants with common diseases and complex traits from the UK Biobank, we identified some overlap with our driver variants. This strengthens the biomedical importance of these variants, which was also confirmed by searching OMIM. In fact, we found that several genes we identified were implicated in inborn errors of metabolism. These findings show that there is a continuum between monogenic disorders, common disease and quantitative variation.
Were there any surprising results?
Yes! Among the associations we found, ACY1 stood out for a couple of reasons. While most genes were associated with only one or two metabolites, we found 7 metabolites associated with ACY1. Its association with N-acetylmethionine also showed a surprising directionality: we found that rare variants in the ACY1 gene were associated with an increased level of N-acetylmethionine, but the same variants were also associated with a reduced level of ACY1 protein. We think that this may be due to a feedback loop, where accumulation of the metabolite causes a reduction in the protein level.
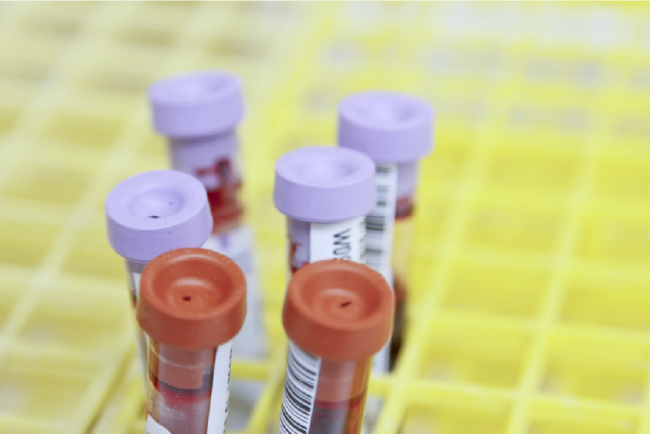
What are the next steps in this work?
We are currently working on another manuscript that will be an extension of this work. Our follow-up study is more comprehensive, and it uses a much larger number of whole genome sequences with a larger and more diverse number of molecular phenotypes. In addition to rare coding variants, which was the focus of this first paper, we will also be able to look at rare non-coding variants. This will allow us to identify possible regulatory mechanisms with potentially large effects on metabolite levels.