How the Open Targets Platform Integrates Gene Burden Analyses
Last year we added a new data source to the Open Targets Platform: Gene Burden. This feature assesses the contribution of rare variation in all protein-coding genes to more common, complex traits.
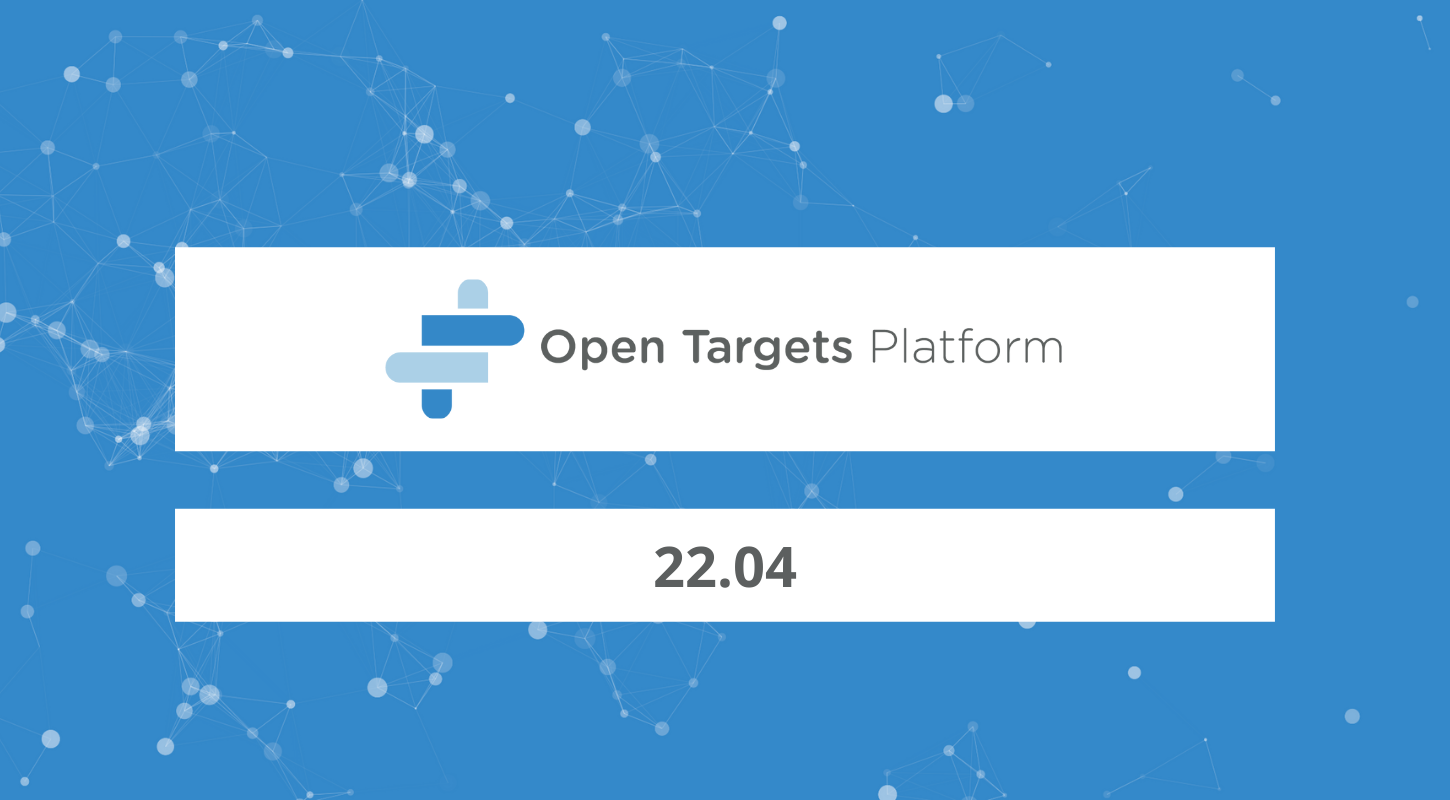
As more patient genomes are sequenced, the genetics community is increasing its ability to detect rarer disease-causing variants and collapse them into mechanistically insightful gene associations. The Platform aims to contextualise this information with other publicly available data to improve on our ability to systematically identify disease-causing genes.
After incorporating various burden analyses on the UK Biobank cohort, we have continued to expand our data sources by including additional disease-specific burden tests. As a result, we have become the reference repository for associations based on collapsing analyses. Studies on other cohorts include:
- the SCHEMA Consortium on schizophrenia;
- the AMP-PD Initiative on Parkinson’s disease;
- the study on metabolites carried out by Lorenzo Bomba;
- and our latest addition: the INTERVAL cohort’s exploration of genes linked with cardiovascular metabolic biomarkers
In this post, we will compare the three primary gene burden resources — Genebass, Regeneron, and the AstraZeneca PheWAS Portal — and discuss their contributions to the Open Targets Platform.
What exactly are gene burden analyses?
Burden tests try to leverage the long tail of rare mutational events that can be captured when sequencing the DNA of large cohorts of individuals. Whether it’s by sequencing the whole genome or only the coding part of it — the exome — burden tests aim to estimate the aggregate effect of rare variants across a candidate region, more often a gene.
To increase the statistical power compared to single-point association studies, gene-based burden testing approaches use qualifying variants within the protein-coding boundaries of genes to evaluate whether there is a significant difference between cases and controls. Burden tests assume that all rare variants in the region are causal and affect the phenotype in the same direction; therefore they excel at capturing signals driven by variants predicted to have a detrimental effect on the protein function (for example loss-of-function variants).
At the end of 2021, the UK Biobank released a new version of exome sequencing data [1], which has enabled the research community to study genetic variation in the exomes of 450,000 participants. This repository of phenotypic information forms a particularly rich resource for drug discovery since it provides an opportunity to understand the consequences of protein-altering variation in a large human cohort and helps to identify therapeutic targets.
In our 22.04 release, the Platform integrated burden tests carried out by the Regeneron Genetics Center [2], and the gene-level PheWAS maintained by the AstraZeneca PheWAS Portal [3], both based on the same UK Biobank data. Our 22.06 release built up on this, including the results of the Genebass consortium in the same UK Biobank exome data [4]. As part of the process to integrate all studies in the Open Targets Platform, all phenotypes were mapped to the Experimental Factor Ontology with the assistance of the GWAS Catalog curation team.
The following section provides an illustration of the variations observed among the three UK Biobank analyses, highlighting the individual strengths of each data source and the overall benefit of analysing them collectively.
Gene burden in the Platform
The analysis by AstraZeneca is based on a subset of approximately 450,000 high-quality exome sequences of predominantly unrelated study participants of European ancestry released by the UK Biobank. These were used to evaluate the association between protein-coding variants with 17,361 binary and 1,419 quantitative phenotypes using variant-level and gene-level phenome-wide association studies.
In contrast, Regeneron and Genebass report a narrower scope in the number of traits studied by requiring a bigger minimum sample size: 3,994 health-related traits (3,702 binary and 292 quantitative) in the case of Regeneron and 4,529 (3,296 binary and 1,233 quantitative) for Genebass. In the Platform, we have selected those gene/phenotype associations that are statistically significant (p-value ≤ 2 × 10-9 for AstraZeneca's PheWAS pPortal, 2.18 x 10-11 for Regeneron, and 6.7 x 10-7 for Genebass), adding up to a total of 27,067 evidence strings.
Key stats
AstraZeneca PheWAS Portal | Regeneron | Genebass | |
---|---|---|---|
Individuals | ~450,000 | 454,787 | 426,370 |
Statistical significance level | 2 × 10-9 | 2.18 x 10-11 | 6.7 x 10-7 |
Genes | 713 | 483 | 1436 |
Binary traits | 870 | 216 | 175 |
Quantitative traits | 234 | 213 | 308 |
Union traits | 370 | 0 | 0 |
Traits mapped to EFO | 431 | 205 | 299 |
Gene-quantitative trait direct associations | 1,518 | 1,168 | 3,093 |
Gene-binary trait direct associations | 875 | 229 | 656 |
Collapsing models | 10 | 10 | 4 |
Evidence | 11,767 | 7,382 | 7,901 |
Ancestries | European | Multi-ancestry | European |
When we analyse the scope of the sources, we see that AstraZeneca's approach includes a broader phenotypic variety, which can be attributed to their focus on covering metabolomic-related traits and other measurements, as well as their inclusion of union phenotypes based on the combination of similar phenotypes.
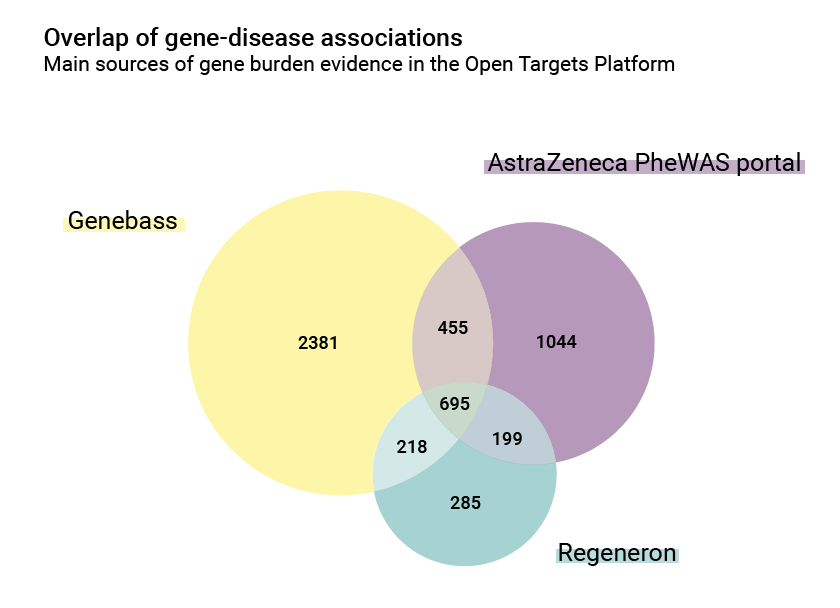
There are also differences in the number of associations: the AstraZeneca PheWAS portal contributes 2,393 gene/disease relationships, while Regeneron and Genebass contributions are 1,397 and 3,749 respectively. The overlap between them is however significant and is a good index of common biology: 695 of these associations are shared.
Although AstraZeneca's analysis covers a greater range of traits, Genebass's results involve a larger number of targets and contributes a significant number of new associations to the Platform. This is reflected in the fact that Genebass has a lower median number of linked traits per gene, at 1, compared to the AstraZeneca PheWAS portal and Regeneron, which have a median of 2. The reason for this difference may be attributed to the nature of the different collapsing methods used in the analysis; let’s take a look at the role of these methods.
So what are collapsing methods?
A collapsing method is defined by the set of filters that extract qualifying variants for the association study between the candidate gene and the trait. Filters focus the analysis on the class of variation that confer risk (loss-of-function) or are protective while filtering out the variants with insignificant biological importance. Therefore, the effectiveness of collapsing analyses mostly depends on optimising the filters.
Commonly used filters include sequencing-based quality metrics, predicted variant effects, predicted deleteriousness, and, most importantly, population allelic frequencies.
The outcome of the test is highly dependent on the choice of the allelic frequency threshold. Some caution is required in its selection, as there must be a balance between retaining rare causal variants and reducing the number of non-causal mutations in the aggregate.
We have integrated 35 different models to aggregate qualifying variants which, though they differ depending on the source of the study, capture an analogous type of information: the prevalence of deleterious mutations across very low frequencies.
In the figure, one can appreciate the role that ultra-rare and very rare variants have on the full association set. To be more precise, protein-truncating variants (PTVs), which often inactivate proteins, are of great importance because they provide direct insight into human biology and disease mechanisms. This illustrates the power of exome sequencing to detect rare and ultra-rare variants that contribute to disease.
For diseases under strong negative selection such as ichthyosis, restricting the analyses to the rarest variants has shown strong enrichments for causal variants.
Direction of effect
The direction of effect provides information about the role of the gene in the disease, distinguishing between risk-conferring and protective mutations in the gene. In this context, we examined how often significant associations across different collapsing models in the same gene have opposite directions of effect for the same phenotype, as this negatively affects the statistical power of the association.
Our analysis reveals that the directions of effect are consistent for associations between continuous and binary traits across different collapsing models and sources, coinciding in 98% and 87% of cases, respectively. An example of a binary trait is hypercholesterolemia, where PCSK9 is known to have a protective role (OR < 1), Similarly, we can also see the protective effect of PCSK9 qualifying variants for an example of quantitative trait (cholesterol measurement) with negative beta values.
We also found that gene–phenotype associations are also significantly enriched for loss-of-function-mediated traits.
Ancestry specificity
Regeneron performed additional collapsing analyses for each major non-European ancestral group. However, no new gene-trait associations were identified from these analyses, which may be due to the low number of non-European participant samples. Wang et al. do report a unique significant association between PTVs in HBB and thalassaemia in individuals of South Asian ancestry within the UK Biobank data [3]. This association is observed when increasing the sample size and performing the same collapsing analysis on the 450,000 individuals, demonstrating that diversity in genomic datasets is important for novel discoveries.
Nevertheless, these populations are still greatly underrepresented in the UK Biobank and additional cohorts will be used to capture the more complex genetic diversity of human genomes.
What is the contribution of Gene Burden evidence to the Platform?
One of the key aims of the Open Targets Platform is to showcase associations supported by an underlying genetic mechanism.
The gene burden evidence provides a different perspective to the existing evidence in the Platform, providing evidence from rare variants that contribute to common traits. While they add only a limited number of new associations (green), the results of the burden tests anchor known associations, and provide a method for unravelling the complex mechanisms of common diseases.
Convergence with Open Targets Genetics
It is of particular interest to make a comparison between the contribution of gene burden with the Open Targets Genetics (OTG) associations, as this is our main repository of common variation.
Looking at the associations shared between sources, we see the greatest overlap with OTG - 52% of gene burden associations are present in OTG (31% for binary trait associations, 56.2% for quantitative traits). This is very consistent with the recent findings of Weiner et al [5], suggesting that SNPs and rare mutations often point to the same underlying biology.
If both rare and common variants in a gene are associated with the same phenotype, this suggests a dose-dependent relationship between the variants and the phenotype.
We also see comparable results when we look systematically at the polygenicity of the burden associations: each trait has a median of 2 exome-wide significant genes associated with the trait. The fact that we see fewer genes involved facilitates following them up functionally compared to common variation.
We are very excited about the contribution this new source has made to the Platform, and we are actively seeking to expand our coverage of genetic diversity by including studies on populations from different regions that shed light on the search for new relevant targets.
References
- 450,000 participant exomes made available today for approved researchers through Research Analysis Platform, UK Biobank, 29 October 2021
- Backman, J.D., Li, A.H., Marcketta, A. et al. Exome sequencing and analysis of 454,787 UK Biobank participants. Nature 599, 628–634 (2021). https://doi.org/10.1038/s41586-021-04103-z
- Wang, Q., Dhindsa, R.S., Carss, K. et al. Rare variant contribution to human disease in 281,104 UK Biobank exomes. Nature 597, 527–532 (2021). https://doi.org/10.1038/s41586-021-03855-y
- Karczewski K.J., Solomonson M., Chao K.R. et al. Systematic single-variant and gene-based association testing of thousands of phenotypes in 426,370 UK Biobank exomes. medRxiv (2022). https://doi.org/10.1101/2021.06.19.21259117
- Weiner, D.J., Nadig, A., Jagadeesh, K.A. et al. Polygenic architecture of rare coding variation across 394,783 exomes. Nature 614, 492–499 (2023). https://doi.org/10.1038/s41586-022-05684-z