Open Targets Platform 22.04 has been released!
The latest release of the Platform — 22.04 — is now available at platform.opentargets.org.
Key points
- New datasource: gene burden analyses from Regeneron in the GWAS Catalog and the AstraZeneca PheWAS portal
- Integration of structural variants from ClinVar
- Additional information from DailyMed drug label text-mining
- NLP classification of why clinical trials stopped
- New data: Gene2phenotype cardiac panel
- Also: renaming Phenodigm, removing the PheWAS Catalog datasource
Key stats
Metric | Count |
---|---|
Targets | 61,524, of which 29,162 are associated with at least one disease |
Diseases | 18,520, of which 15,316 are associated with at least one target |
Drugs | 12,854 |
Evidence strings | 13,829,174 (see the Community for a breakdown by datasource) |
Associations | 7,541,360, of which 2,230,103 are direct associations |
Gene burden analyses of UK Biobank exome sequencing
We are particularly excited to announce that this release features a new datasource named “Gene burden” that will incorporate the results from the aggregation of rare and ultra-rare variants at the gene-level.
The first release of this datasource includes the results from two gene burden analyses performed on exome sequencing of over 450,000 individuals sampled by the UK Biobank and recently published in Nature: Wang et al. 2021 and Backman et al. 2021. The analysis, performed by colleagues at AstraZeneca and Regeneron, respectively, aims to identify the accumulation of rare genetic variants that would explain binary or quantitative traits. The large number of samples and phenotypes analysed provides a systematic picture on the effect of loss-of-function mutations across the genome.
The Platform incorporates the two complementary analyses in the context of all publicly available genetic information — either common diseases (Open Targets Genetics portal), rare diseases (ClinVar, GEL PanelApp, Orphanet, ClinGen, Uniprot variants) or somatic variation (ClinVar somatic, Cancer Gene Census, IntOgen).
The breadth of binary and quantitative phenotypes analysed complements some of the previously pinpointed genes. For example, PCSK9 was already associated with apolipoprotein B measurement using GWAS in the Genetics Portal via two intronic variants. The inclusion of the UK Biobank gene burden data now allows us to better understand that loss-of-function mutations in PCSK9 are significantly associated with a decrease in circulating apolipoprotein-B.
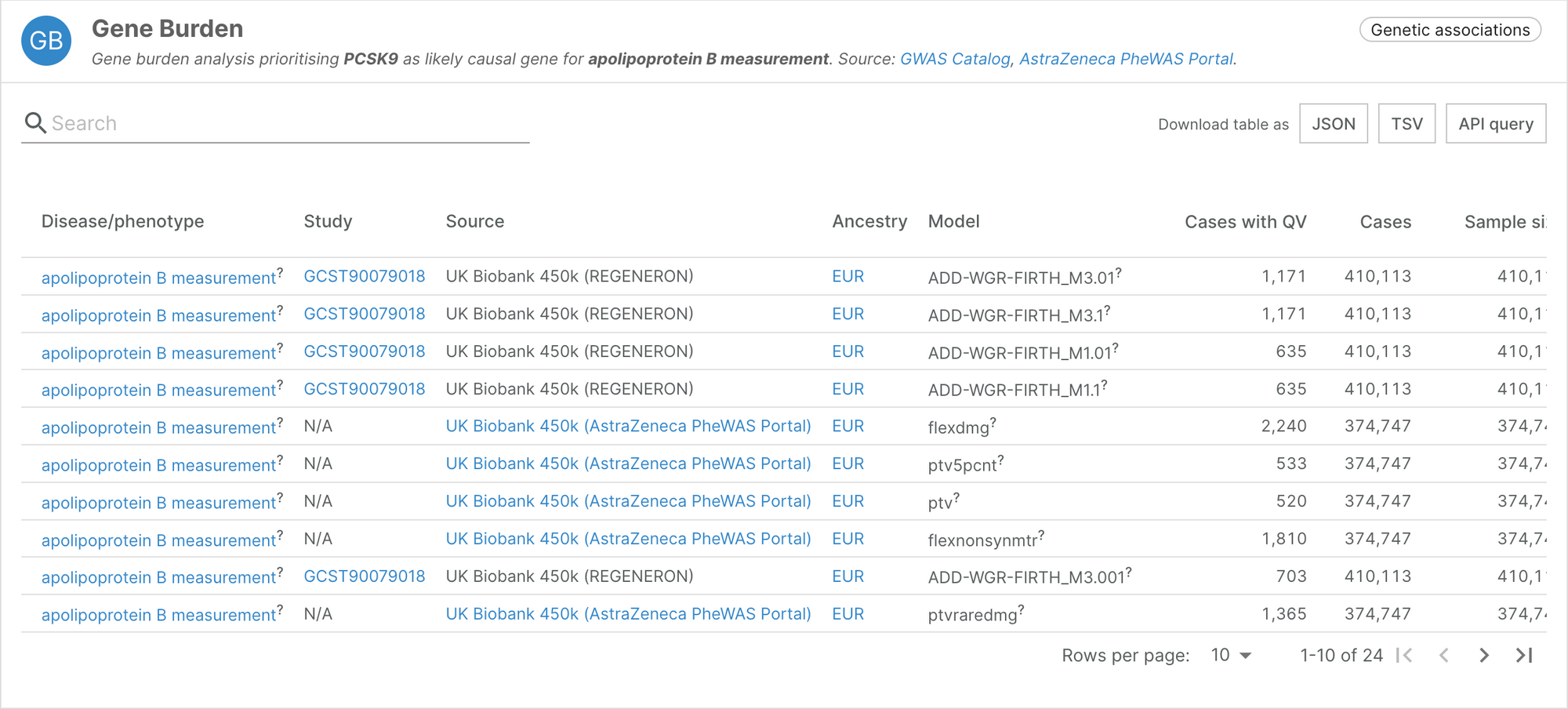
For the first time in the Platform, this widget collates genetic information in different ancestries, such as the protective effect of anomalous erythrocyte volumes associated with HBB in regions affected by malaria. In the future, we hope better access to genetic information in more diverse populations will unblock the development of new therapies.
Thanks to the GWAS Catalog, the raw information is available for download (explore Regeneron’s summary statistics). For more in-depth analysis of the AstraZeneca analysis, please visit the AstraZeneca PheWAS portal.
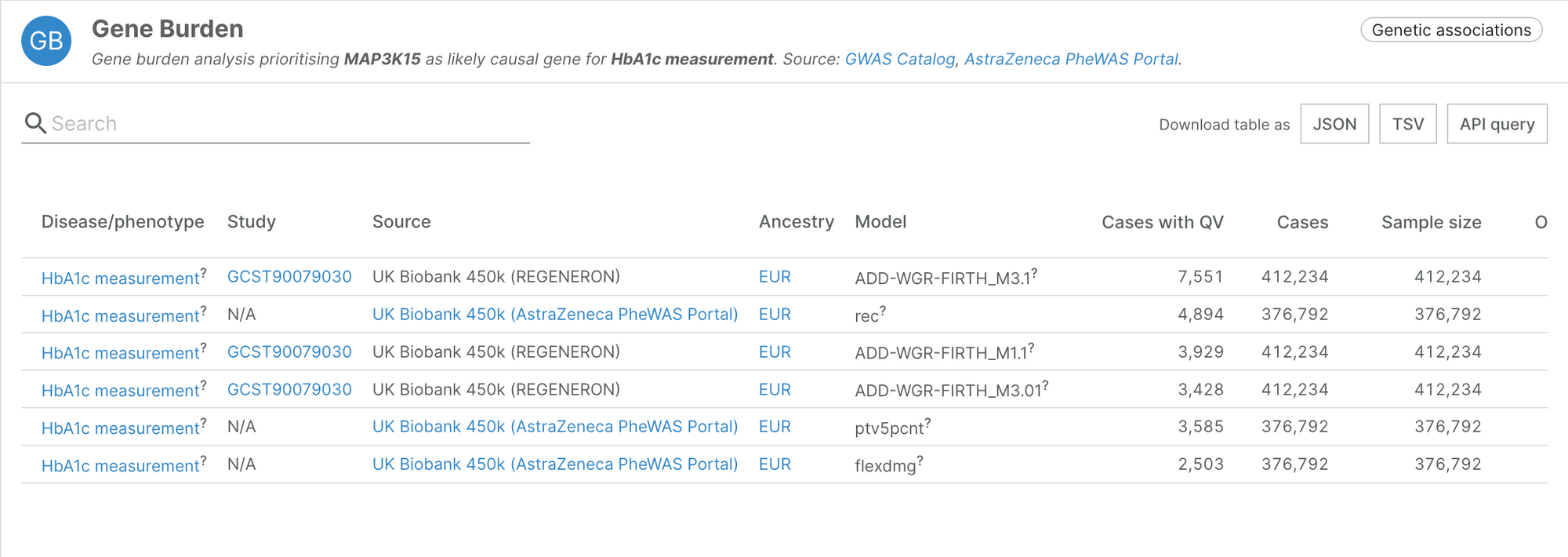
Integrating structural variants from ClinVar
Structural variants represent more complex rearrangements of the genome such as large copy number variants, inversions, translocations, and more. The Platform now integrates information on such variants through the European Variation Archive (EVA). Genomic location for single and complex variants are displayed in HGVS format, as reported directly in ClinVar.
The HGVS notation is better suited for structural variants, and is included alongside our other notations such as rsID.
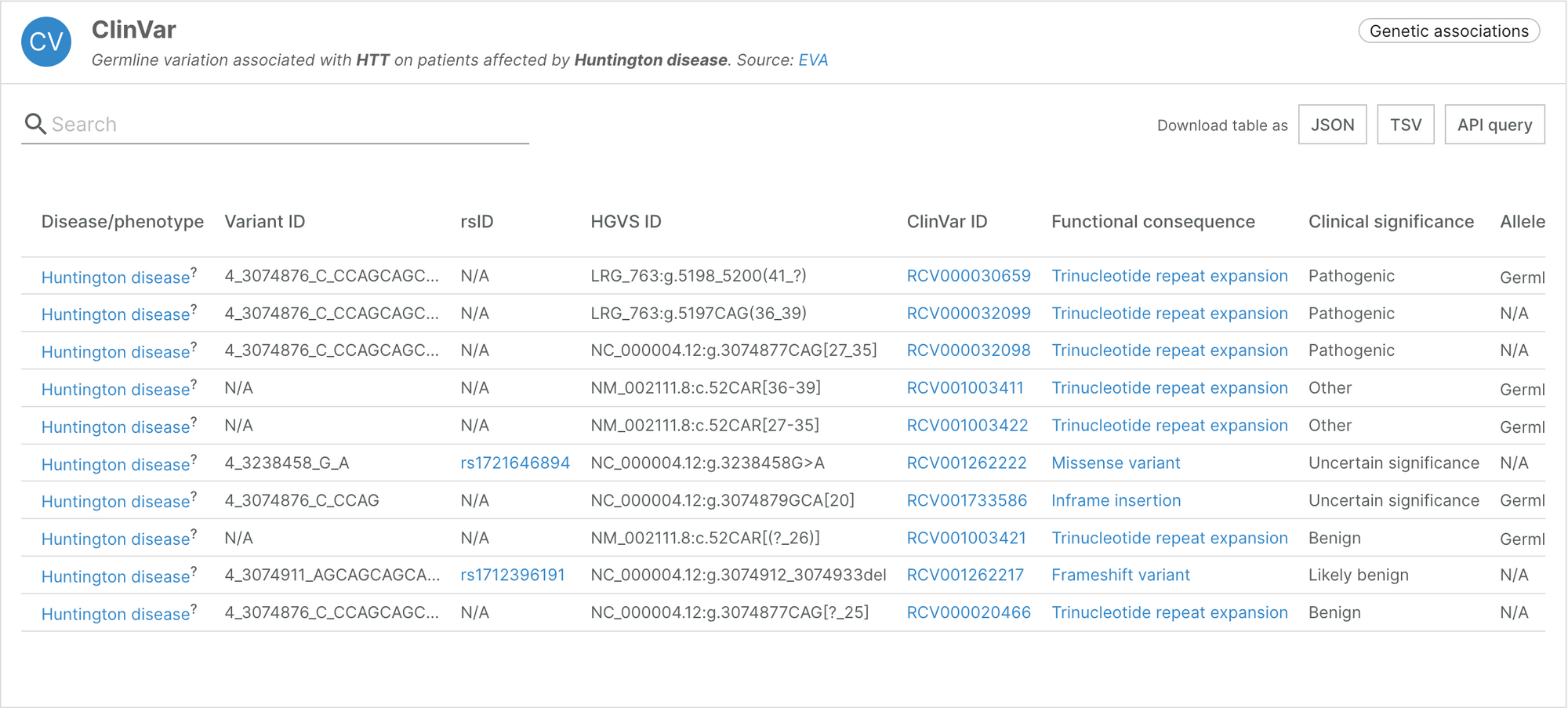
Machine learning-based text mining of drug labels
In this release, we have integrated the results of a text-mining strategy developed through Open Targets to extract drug indications from drug labels.
DailyMed is a free and open access public resource operated by the National Library of Medicine, which provides access to descriptive drug labels that define the indications for all FDA-approved drugs. Using a machine learning approach for natural language processing, drug indications were extracted and mapped to EFO terms, and their associated drugs were mapped to ChEMBL compounds. This has contributed to a significant increase in the number of evidence strings we generate using ChEMBL. In total, the Open Targets Platform integrates over 90,000 references from ChEMBL to create 594,375 evidence strings.
This update is part of the latest release from ChEMBL (ChEMBL 30). The full details and additional features of this update can be found in ChEMBL’s release notes.
NLP classification of reasons why clinical trials stopped
When browsing the target-disease association evidence available in our ChEMBL widget, users will now be able to see the reasons for which clinical trials were stopped early, classified into 17 broad categories. You can find this information by hovering the tooltip next to a trial’s status.
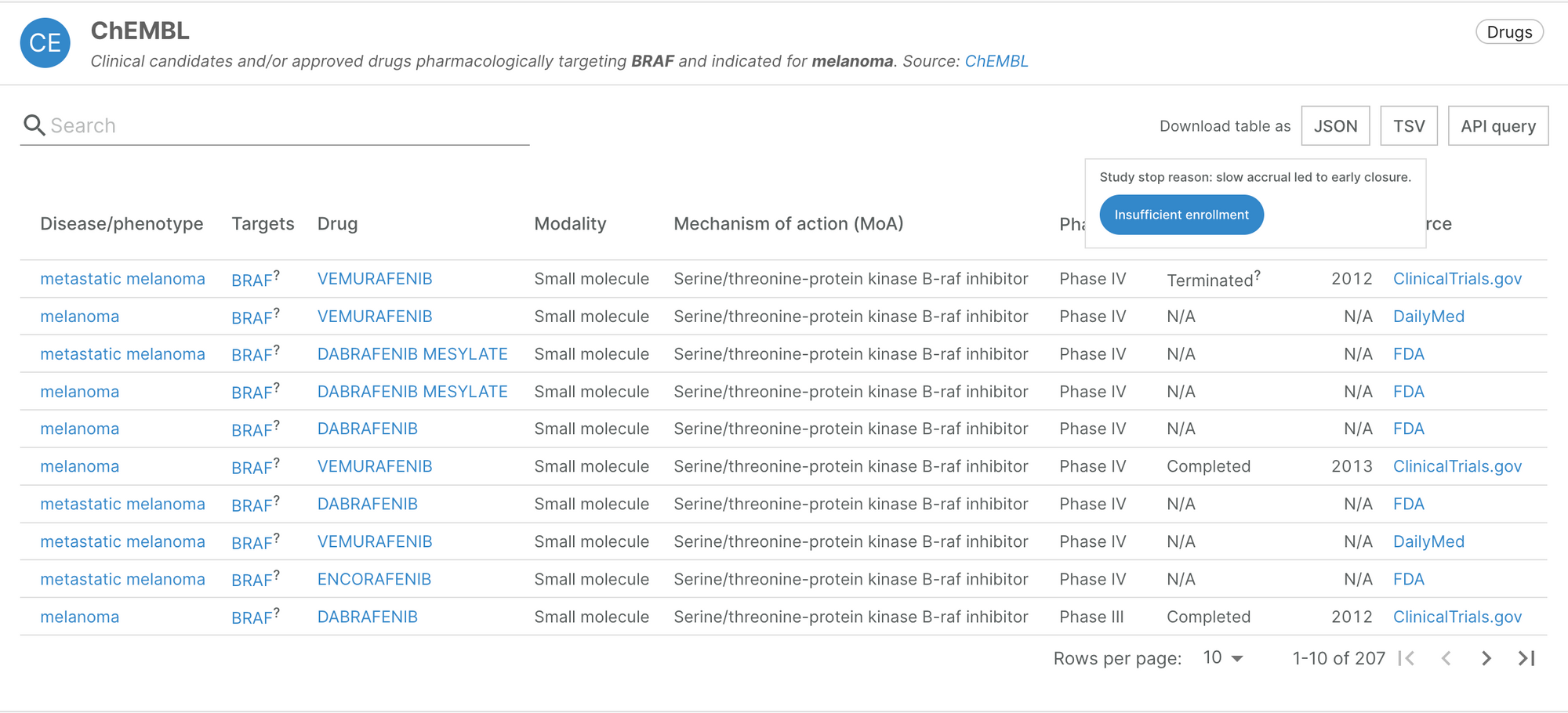
This data is provided by an Open Targets project led by Olesya Razuvayevskaya. The project used Natural Language Processing (NLP) to classify the recorded reasons for which clinical trials were stopped before their endpoints were met, using data from clinicaltrials.gov. ClinicalTrials.gov is a database of publicly available information on clinical studies from around the world. Notably, it provides investigators with a free text field to describe the reason for which their trial was terminated, suspended or withdrawn. The machine learning classifier analyses this free text response to categorise the trial’s stop reason.
The classification provides additional context to the trials, and includes negative reasons (e.g. safety or efficacy concerns), neutral reasons (e.g. business or administrative) and positive reasons (e.g. success). This is reflected in our scoring: trials that have stopped due to negative reasons or because of safety and side effects concerns are down-weighted.
In total, this work provides an additional 55,308 pieces of evidence, from the classification of 8,044 clinical trials.
New Gene2Phenotype panel
Earlier this week, Gene2Phenotype added an additional panel for cardiac diseases, selected by manual curation. This brings in 54 new pieces of evidence, supporting and increasing our confidence in 47 target-disease associations.
For more information about the panel, take a look at Gene2Phenotype.
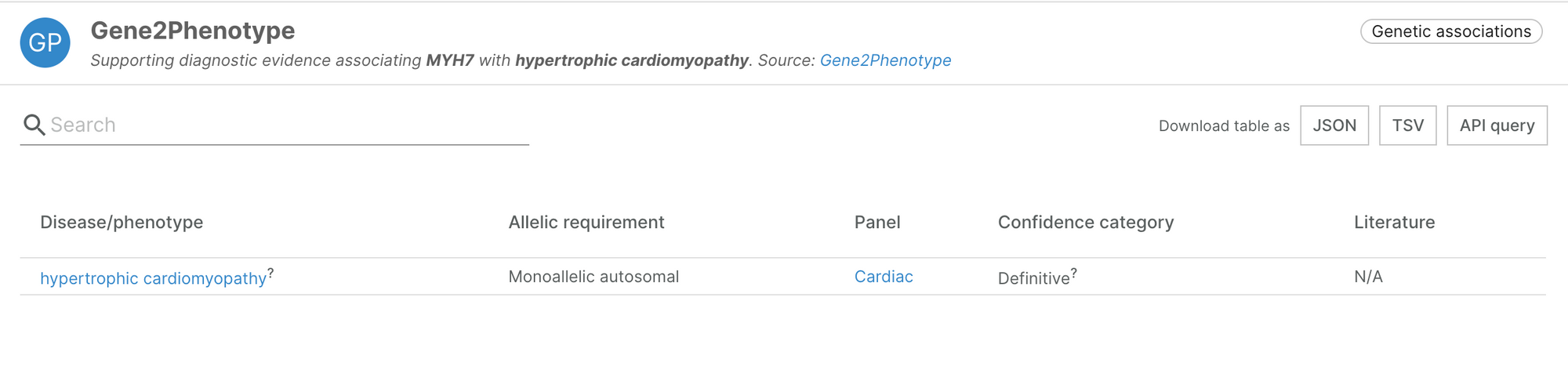
Additional changes
Our ‘Phenodigm’ data source has been renamed to IMPC
The International Mouse Phenotyping Consortium (IMPC) is a worldwide collaboration between 21 research institutions to elucidate the function of every protein-coding gene in the mouse genome by systematically knocking out each gene and inferring its function through subsequent physiological tests.
Phenodigm is the name of the algorithm that establishes the links between mouse phenotypes and human disease phenotypes, whereas IMPC is the name of the service that maintains the data. This change aligns with the way we’ve named our other datasources.
Removing a datasource: PheWAS Catalog
As the data contained within the PheWAS Catalog is static and now out-of-date, we made the decision to remove it as a datasource. Considering the significant overlap with the data we ingest from the UK Biobank and FinnGen through the Genetics portal, we do not expect many changes to target-disease associations in the Platform.
Questions or comments? Let us know what you think of our updates on the Open Targets Community or on Twitter.