Q&A with Lucy Sibbring, an intern with the Open Targets data team
Lucy Sibbring completed a two-month internship with the Open Targets data team.
With a background in biochemistry and bioinformatics, Lucy was interested in exploring how bioinformatics was applied to real-world healthcare data. Her project investigated the cell-specific expression of genes from single-cell human atlas datasets from the Tabula Sapiens Consortium Atlas. She sat down with us to talk about her project and her experience at Open Targets.
Why did you choose to do an internship with Open Targets?
I just graduated with a degree in Bioinformatics, and I was interested to see how the skills I learned could be applied. I wanted to see what kind of roles were out there for bioinformaticians, what kind of sectors I could go into, and the type of work it involved. What does office life look like for a bioinformatician?
My Master’s was a lot of beginner coding, and we would learn with example datasets, which are very clean, almost perfect — great for learning, but not very indicative of what it might be like to work with real data, particularly biochemical and biomedical data, which is where I would like to work. So I searched for possible internships in this sector, and came across Open Targets.
I found the Open Targets platforms were simple to use, and I liked the way you integrate the data and display it prominently, so I wrote to ask whether you had any opportunities, and now here we are!
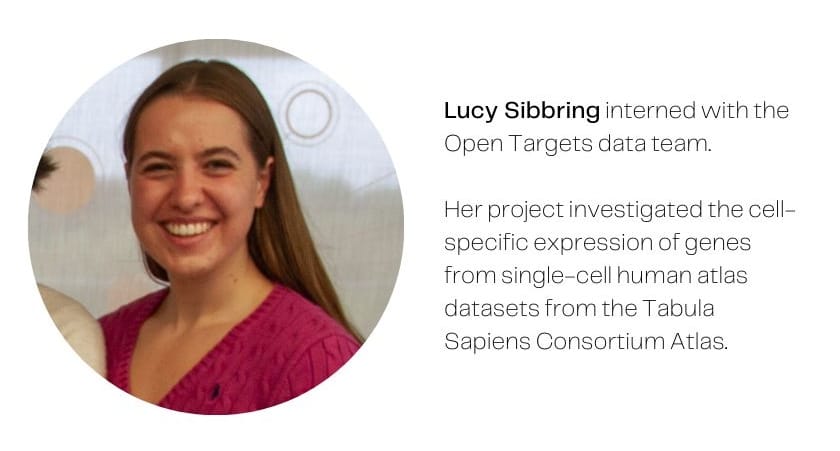
What did you work on during your project?
My project was a look into single cell data, which is when you sequence individual cells rather than sequencing tissues in bulk — this gives you a much more precise view of how cells in an organ are behaving and interacting at a given time, or even over time. You can see how gene expression changes in specific cell types or even individual cells, and get a much finer picture of how a disease or a treatment might impact the biology of a tissue. Open Targets actually has some research projects in this area.
The Open Targets Platform uses quite a lot of bulk RNA expression data, to inform on target expression profiles and disease associations, and the team is currently investigating the best way to integrate single cell expression data as well.
I was focusing on one particular large single cell study, Tabula Sapiens, and looking at the sorts of trends we see across the gene, across different cell types and different tissues. We wanted to make comparisons to the available bulk sequencing datasets, to align this new data with what is already on the Platform. We would expect to see that the average gene expression is similar across bulk and single cell datasets, giving us a rough baseline for gene expression. Once you have a baseline, you can tell whether gene expression in a particular cell or cell type is unexpected.
I also took a look at the specificity and distribution of gene expression: are the genes expressed in just one cell type or across a number of cell types, is it more highly expressed in one tissue than another? These kinds of questions help in target prioritisation.
Personally, I quite like working with numbers. So I find it really satisfying to work with these dataframes with expression count data, analysing them to show a trend or a certain pattern, summarising the analysis, and generally taking something that looks quite chaotic and overwhelming at first and boiling it down to a few simple facts of summary statistics. I like that I have the ability to turn a big dataset into something manageable, and able to present a clear and concise summary of what’s going on.
Was there anything surprising about your results?
This comparison highlighted a lot of anomalous or confusing results in the single cell dataset. For example, insulin is a gene that we expect only to be expressed in the pancreas beta cells, but I found really high counts for insulin expression in some natural killer immune cells, which was very surprising. Ultimately this means we need to be careful about how we pre-process the data.
You have to think very critically about how the experiments were done and what aspects might affect the results, and whether the results you are seeing are real or an artefact of the experimental protocol, particularly for something like this where you’re looking at counts for each gene in each cell. I’m not very well versed in wet lab procedures, and it can be hard to understand exactly how an experiment was conducted when you didn’t do it yourself.
What wast the most challenging part of your project?
I had a couple of struggles with the coding, purely because I only learnt Python last year, so it’s still a learning process. Getting to grips with that — for example how to adjust the tables so that I could apply a function that had already been developed — that was sometimes a struggle.
I like getting things right, so when something doesn’t work and keeps on not working and not working, that’s very frustrating for me. But I feel like that makes it so much better when you do get a solution and it does work. So I would say it’s one of the biggest struggles but also one of the biggest satisfactions you get from doing this kind of thing.
What are your key takeaways?
I really enjoyed myself over the past couple of months. I was originally meant to be working in a hybrid pattern, but I found that working in the office every day was a lot better for me socially. I was able to be more integrated in the team, meet and talk to people. I would not recommend going to the office on a Friday, there is no one [laughs].
One of the things I wanted from this internship was to experience office life — I’ve been a student up until now so the idea of working in an office is elusive, particularly for a scientific team. And actually it’s quite a collaborative environment, which I quite like. The team makes that very easy, and I found that the executive team is very integrated with the normal working team, and there is a community feel to it. The fact that you do things like the Wednesday social when we carved pumpkins — that’s brilliant!
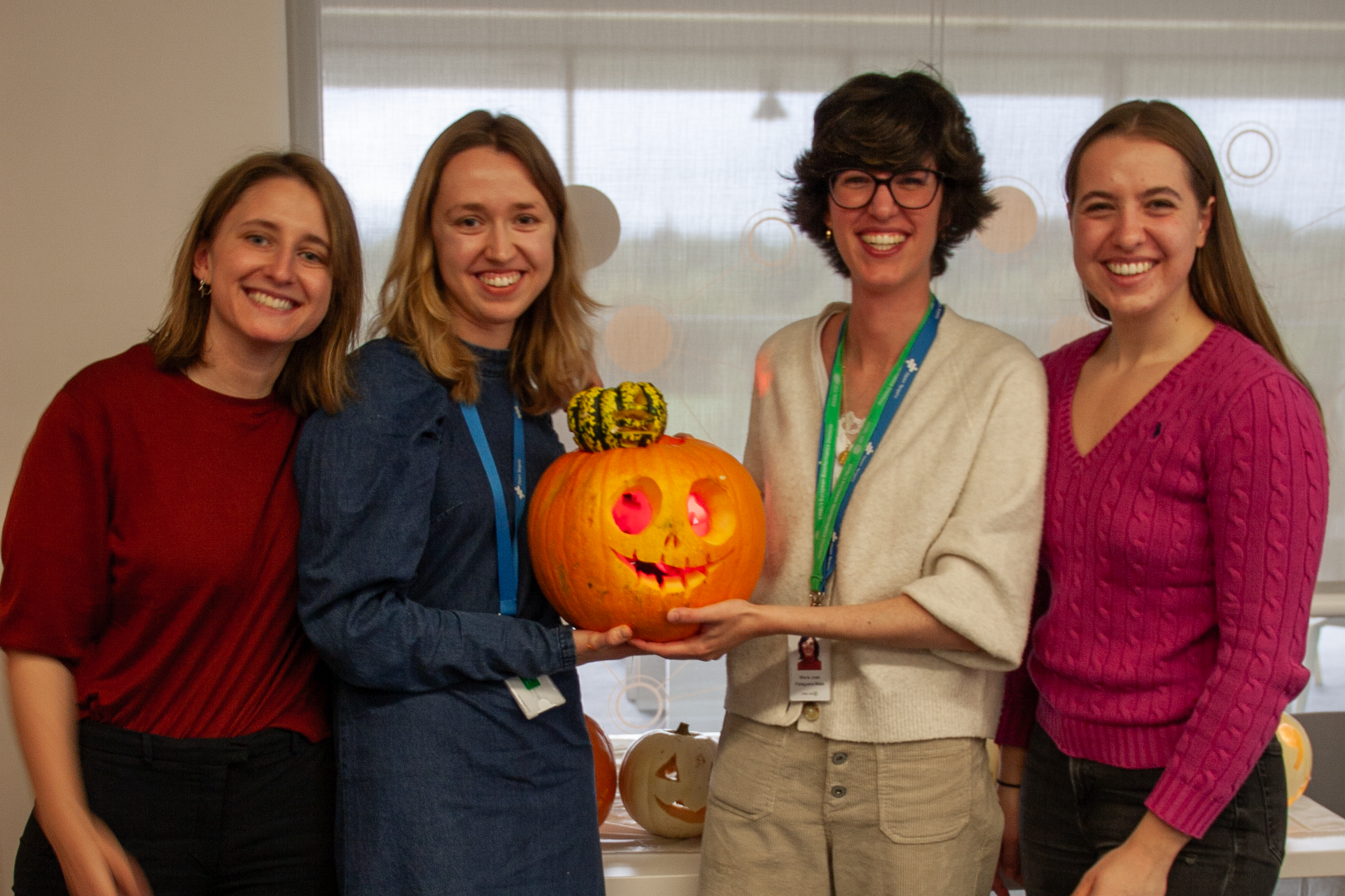
What’s next for you?
In the short term, I have a new job in commercial data analysis back in Stoke. I’m then going to go travelling for a year, which has been one of my bucket list items for a while.