Integrating pharmacogenetics data: a new lens for target prioritisation
In December 2023, we introduced the pharmacogenetics widget in the Open Targets Platform, which brings in data from PharmGKB on the influence of genetic variation on drug responses.
PharmGKB is a rich knowledge base of curated annotations and information about gene-drug-disease relationships, including drug pathways and clinical guidelines as well as the genotype-phenotype associations highlighted in our first version of the widget.
What's new?
Pharmacogenetics examines the link between genetics and drug response, helping in the prioritisation of drug targets that minimise the risk of adverse effects.
The new pharmacogenetics data allows you to find pertinent pharmacovariants on the drug page, identify variants within genes that may influence drug responses on the target page, and gain insights into adverse drug reactions through the target safety widget.
In this post, we’ll be diving deeper into how we processed and enhanced the data to be integrated into the Platform.
Metric | Count |
---|---|
Genotype/drug response relationships | 3,8391 |
Relationships with variant-specific prescribing guidance in clinic | 5,397 |
Drugs with pharmacogenetics data | 646 |
Targets with pharmacogenetics data | 1,126 |
Target/adverse drug response relationships | 2,014 |
Establishing a data model
Our first task in processing PharmGKB data was to understand the contents and come up with a data model that captures the most important facets of their clinical annotations, while also being flexible enough to adapt to other possible pharmacogenetics data sources and integrate with data elsewhere on the Open Targets Platform.
Variant and Haplotype Representations
One of the challenges we faced was how to identify variants in PharmGKB records. There are a number of variant identifiers that have strengths and weaknesses for different use cases, but our priority was to harmonise the data as much as possible with other Open Targets datasets. This was further complicated by the fact that many clinical annotations in PharmGKB are specific to a genotype rather than a variant, which is different from other clinical variant databases like ClinVar which tend to be variant-specific. To preserve this specificity, we adapted the VCF-style variant identifier used elsewhere in the Platform to work for genotypes as well.
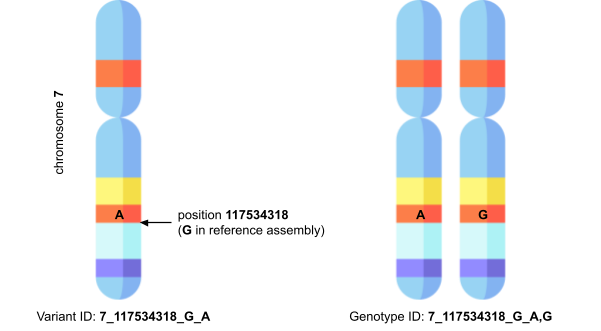
For example, where a variant would be identified as 7_117534318_G_A, indicating single nucleotide polymorphism G>A at position 117534318 on chromosome 7, a genotype might be identified by 7_117534318_G_A,G, where the (A,G) indicates the two alleles in the genotype. This genotype identifier retains the human- and machine-readability of the variant identifier, and the two are clearly compatible with one another in the sense that you can decompose the genotype ID into two variant IDs.
Having these genotype and variant identifiers with coordinates also helps us augment the information in PharmGKB with functional consequences, by passing this information to Ensembl’s Variant Effect Predictor. This provides extra biological context not present in PharmGKB, such as whether the variant is in a coding, non-coding, or regulatory region.
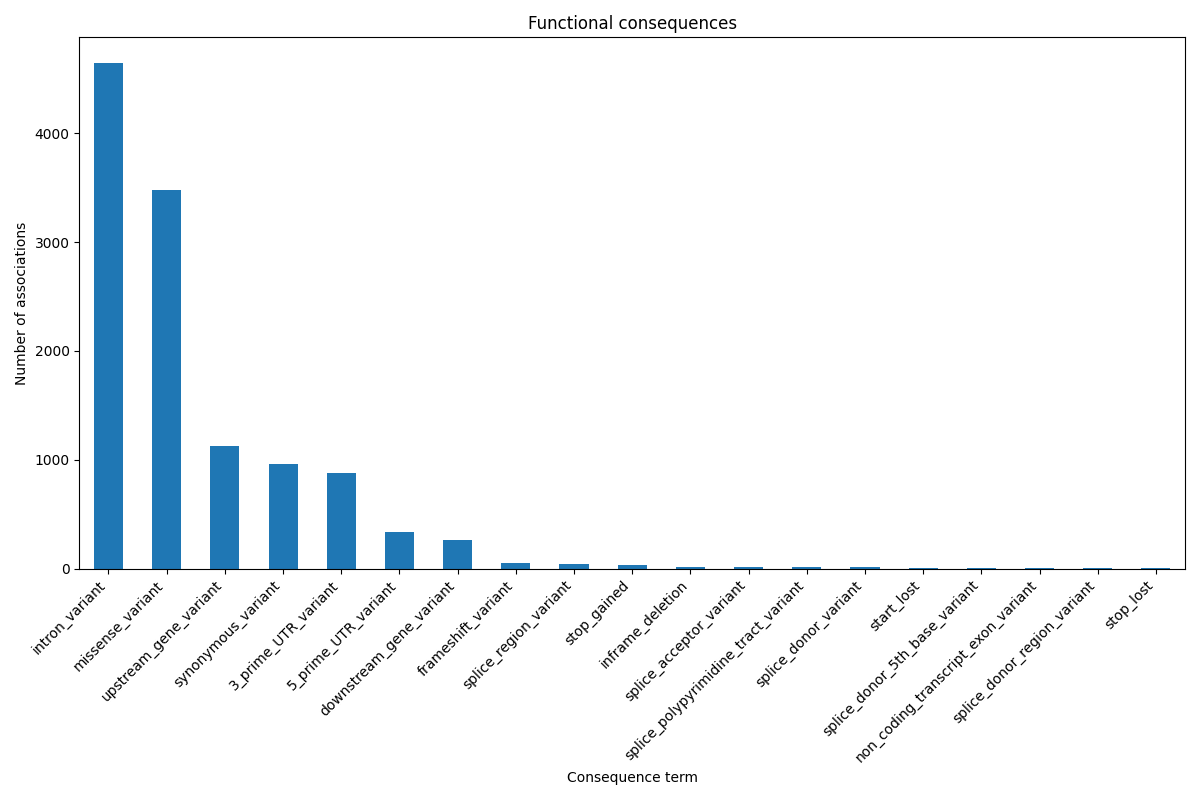
Aside from variants and genotypes, another important part of the pharmacogenetics data is annotations for named alleles (also sometimes called star alleles). These are commonly used in the pharmacogenetics community to refer to haplotypes of important genes, such as the CYP system. These names are convenient shorthands for frequently studied and cited haplotypes, particularly so when the haplotype can consist of many individual variants. They’re also critical for settings such as the HLA complex, where it’s infeasible to call precise variants but necessary to refer to different alleles in standardised ways.
For these records, we identify the haplotype simply by its name, such as CYP2D6*2 or HLA-B*15:02, as well as linking to PharmGKB’s description of the haplotype to provide more detailed information. We provide the rest of the data, including phenotypes and genes, in exactly the same way as for the single variant records. This means all the data for both haplotypes and single variants can be viewed together for a particular gene or drug, using the nomenclature that the pharmacogenetics community is already accustomed to.
Enriching Phenotype Descriptions
While PharmGKB provides a concise phenotype linked to the genotype, their format presents limitations to pulling out the phenotype directly related to the genotype-drug response association. For example, we don't have a phenotype in 15% of the data. When we do, it could reference either the underlying condition of the patient (for example, neoplasm) or, conversely, the resulting outcome phenotype of the pharmacogenetics association (for example, drug toxicity). So to fully understand the relationship, we frequently found ourselves needing to consult the description of the genotype effect for additional context.
To adapt PharmGKB's rich knowledge for our platform, we leveraged OpenAI's text generation endpoint. We processed over 15,000 different phenotype descriptions, extracting the specific drug response outcomes from the clinical annotations. This enriched representation complements PharmGKB's curated text, enabling us to highlight the specific drug outcomes for our users.
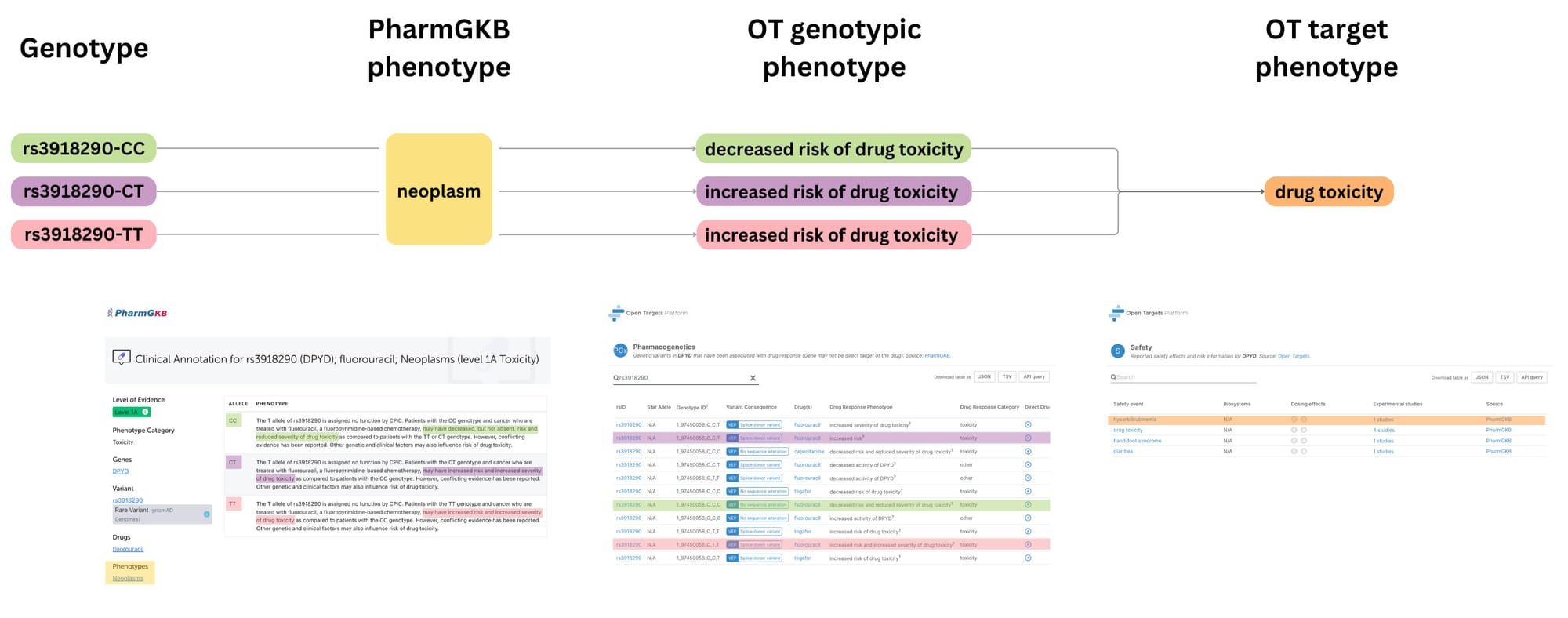
Target Prioritisation
By analysing genotype-phenotype relationships, particularly those related to adverse drug reactions, we can identify targets that may be more or less risky to modulate from a safety standpoint, using genetic variation as a proxy for target modulation. This information can be used to prioritise targets with a lower likelihood of causing severe side effects in specific patient subgroups.
One of the key challenges in leveraging the pharmacogenetics evidence for target prioritisation is that the data is specific to genetic variation. To pinpoint safety liabilities at the target level, we distilled these genotype-specific effects into a target-centric view of potential safety liabilities, as we illustrate in the diagram above. This process removes the "directionality" associated with genotypes (i.e., whether a specific variant is linked to increased or decreased drug response) and focuses on the overall safety profile of targeting a particular molecule.
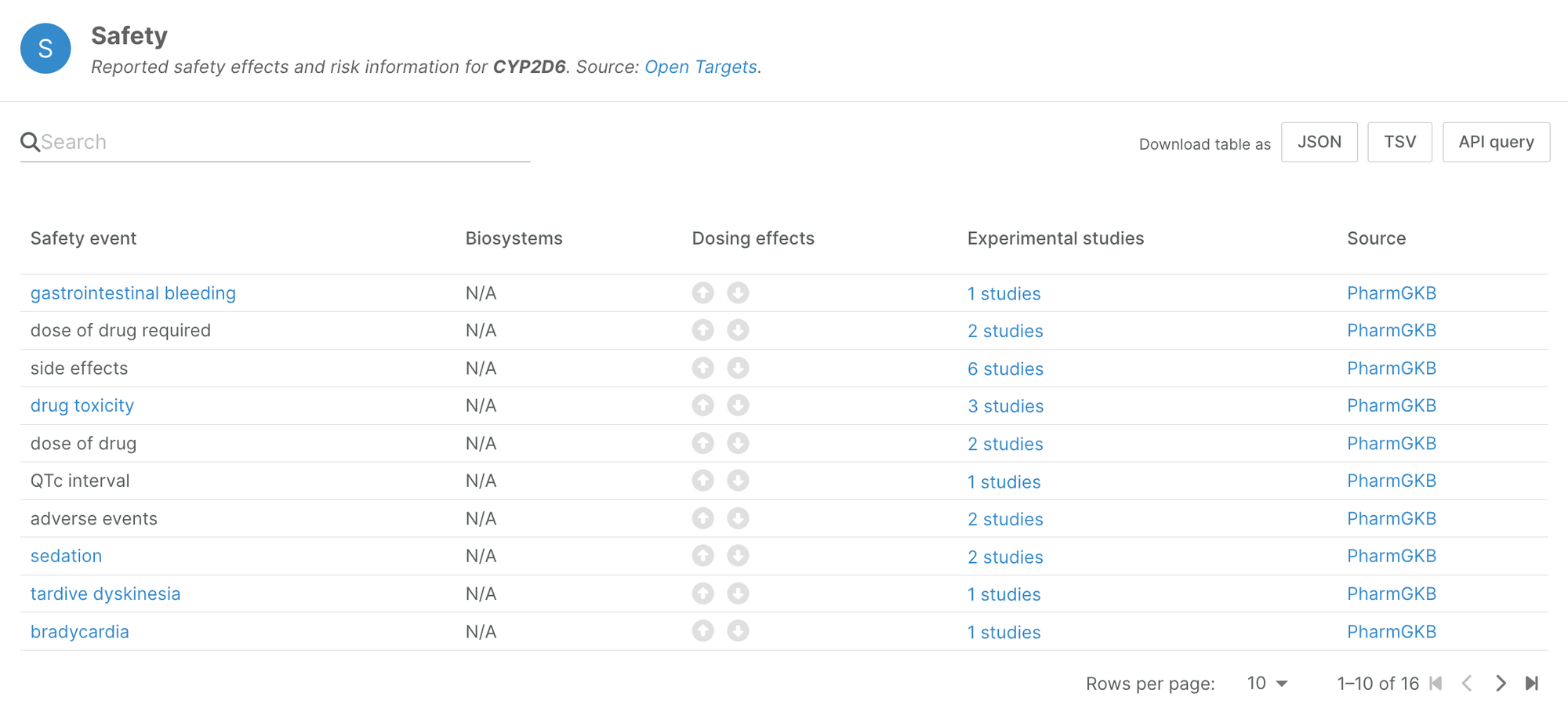
This novel data source has unveiled safety information for 541 genes previously unreported in other datasets, enriching our understanding of drug responses and associated risks. For instance, a comprehensive analysis of pharmacogenetics evidence for CYP2D6, a key enzyme involved in drug metabolism, revealed 16 distinct safety events, each tied to specific star alleles and their implications for drug toxicity.
It's important to remember that these safety liabilities must be interpreted within the context of real-world drug use. The data reflects the experiences of actual patients and can inform clinical decision-making. This high-level aggregation serves as a gateway to delve deeper into the pharmacogenetics widget for insights into specific genotypes and their directional influence on drug response.
PharmGKB is the largest repository of pharmacogenomics guidelines and we are thrilled to integrate this powerful new lens to inform target selection into our Platform. There is still some work to be done, such as fine-tuning the phenotypes for EFO mapping or properly annotating named haplotype alleles for integration with the remaining data, since predicting functional consequences for haplotypes is a significantly more difficult problem.
We’re excited about this first step and for the future directions we will take. We invite the community to explore this integration, your feedback will be invaluable to us!