Building the eQTL Catalogue: a Q&A with Kaur Alasoo
Kaur Alasoo is a group leader at the University of Tartu, in Estonia. He spearheaded the creation of the eQTL Catalogue, the largest compendium of uniformly processed eQTL summary statistics and fine-mapping results.
They have just published their methods in Nature Genetics, so we sat down with Kaur to find out more about how the project came together.
Expression Quantitative Trait Locus (eQTL): an expression quantitative trait is the amount of mRNA transcript of protein. Genomic variants that explain variations in the expression levels of nearby genes are called eQTLs.
How did the eQTL Catalogue come about?
I was first introduced to Open Targets through my PhD with Dan Gaffney at the Wellcome Sanger Institute. Soon after my PhD ended, Dan Gaffney, Daniel Zerbino from EMBL’s European Bioinformatics Institute (EMBL-EBI) and I submitted a proposal to build a pipeline to process and store human eQTL data. Our project was funded, leading to the creation of the eQTL Catalogue.
Rather than simply gather the summary results from all studies in one place, we wanted to get access to the raw study data in order to re-analyse everything with our own workflows. And I remember there was some — probably justified — scepticism from the Open Targets directors: did we have to do it the hard way?
Once we started working on this, we soon realised that we couldn’t have done it another way. There were too many differences between the processed datasets, making them very difficult to compare. Reprocessing the data is complicated, but the end data is much cleaner and easier to use. Researchers can take this data to perform colocalisation studies or lookups, without needing to spend weeks or months finding and harmonising the data.
What is it like working on a project that is split between Estonia and the UK?
Honestly, it’s been one of the best collaborations I’ve ever been in. My team in Tartu applies for access to the individual datasets, then carries out the data processing. Now that we have robust workflows that can easily be rerun, it’s relatively easy to integrate new datasets.
Once the data has been processed, it no longer contains personally identifiable data, and can be shared publicly. We transfer the data to EMBL-EBI, where James Hayhurst makes the data available on the FTP.
James also set up an API to enable users to query specific variants or genes across all the datasets. This was quite challenging: in the previous release, the summary statistics represented around 7 TB of data, the equivalent of 3,500 HD movies. This has only increased in the current release. Building an API that is able to efficiently query a database of that scale is no small feat.
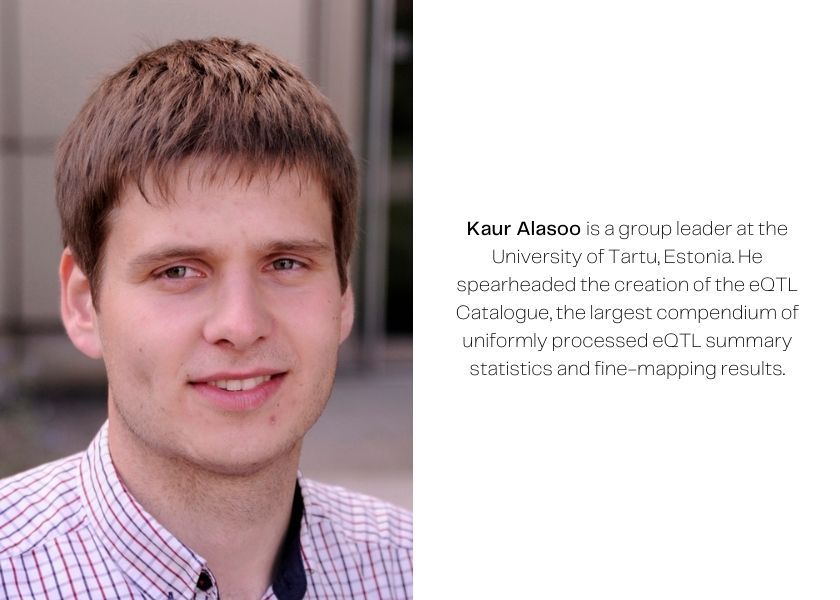
Would say that was the most challenging aspect of the project?
I’d say that the most challenging part of running the Catalogue is getting access to individual raw datasets, because they are hosted in very different repositories, each with their own data sharing systems and portals. Sometimes, as with the NCBI’s database of Genotypes and Phenotypes (dbGaP), the application process is standard. Other repositories are less organised, and we sometimes have to wait 6 months to get an answer to our emails. It is particularly frustrating to go through this whole process only to realise that the data is either not available, or not available for this purpose.
In fact, the process of collecting the raw data is so onerous, that’s probably why we have no direct competitors. We have now processed data from 29 publications, the result of three and a half years of work.
How do you choose which datasets to integrate?
When we submitted our proposal to Open Targets, we made a list of priority datasets based on what our partners were looking for. We’ve integrated most of the bulk eQTL datasets on the list, so we’re now trying to be as comprehensive as possible, and are applying for all the datasets we know of.
One issue with data collection is that we cannot always access individual level data. For example, the consent obtained from the participants sometimes precludes researchers from sharing individual genotypes. And though having individual data in one place makes it easier to process uniformly, it’s not a strict requirement. Therefore, we are planning to move towards a federated model of data analysis, in which we send our workflows to other groups. They can then process their data using our methods, and send us the summary results. Another advantage of this approach is that we would no longer need to carry the cost of storing the data locally. Unfortunately, the standards and technologies for this to happen are not yet mature enough for this to be completely frictionless.
For more information on the eQTL Catalogue’s plans for federated analysis, see https://www.ebi.ac.uk/eqtl/Federated_analysis/.
What’s next for the eQTL Catalogue?
Since we submitted the paper, we have integrated another 8 datasets, bringing the total number of studies we’ve processed to 29. The Open Targets Genetics team is working on integrating these new datasets into the next release of the Genetics Portal.
We are also developing new workflows to integrate single-cell eQTL datasets. The first such studies have been published, including a recent Open Targets project which performed population-scale profiling across dopaminergic neuron differentiation, and it’s equally as important for these to be uniformly processed and made available.
Beyond that, there are other promising data types, such as chromatin and protein data, for which we would also need to develop new workflows. I should say, it’s not that we want to do everything — if somebody else did the work of the eQTL Catalogue, we would be very happy!
You’re very active on Twitter — what role do you think social media has in science?
Twitter is my main source of new interesting papers, and I find myself engaging in more and more scientific discussions. For example, a few weeks ago, Chris Wallace, Masahiro Kanai and I debated how best to use fine-mapping results form the eQTL Catalogue with Chris’ colocalisation method.
I also recently had a discussion with Eric Fauman about some associations he had found, showing that the effect size of a variant attenuates the further you move away from DNA. Eric gave the example of the rs3819817 variant, an eQTL for the HAL gene. The eQTL Catalogue shows that this only overlaps three fine-mapped credible sets, all of which are eQTLs only for HAL and only in skin. The same data can now also be explored in the FiveX browser.
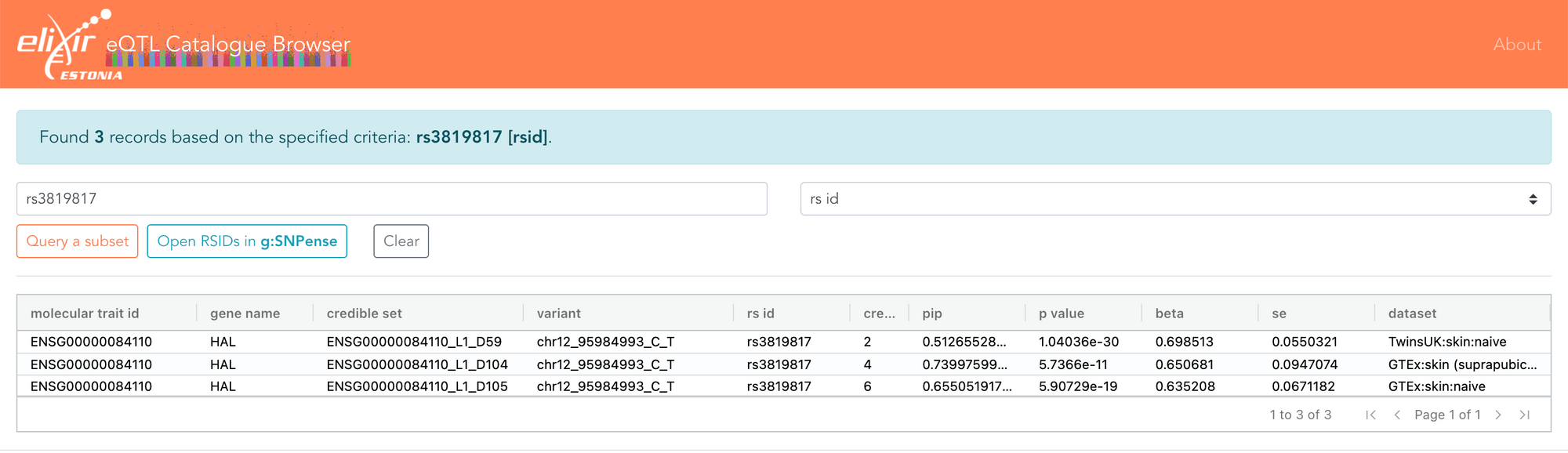
Fine-mapping helps you estimate the probability of a variant being causal, and in this case, rs3819817 has a 51-74% probability of being causal for the HAL gene. It is also the variant most strongly associated with HAL expression in skin.
HAL is an enzyme that converts histidine to trans-urocanate, a metabolite involved in skin pigmentation. Eric’s example showed that if you carry this variant, you have higher levels of trans-urocanate, lower levels of vitamin D, fewer sunburns, you tan more easily, which means you are less likely to need sunscreen. It is satisfying to see when eQTL analysis points to the correct gene (HAL) in the relevant tissue (skin).
These kinds of discussions can foster new collaborations, or allow you to get rapid feedback on your work.
This is one of the figures from my FinnGen talk yesterday. All associations are public - either UKBB, GTEx or this paper: https://t.co/RmgGc8TtLD
— Eric Fauman (@Eric_Fauman) March 17, 2021
Same idea, effect size attenuates as you move further from DNA, all the way from expression to human behavior pic.twitter.com/08UoB1dqW2
The eQTL Catalogue team is currently looking for collaborators who would like to contribute their datasets in a federated manner. Get in touch with them at eqtlcatalogue@ebi.ac.uk.
Citation
Kerimov, N., Hayhurst, J.D., Peikova, K. et al. A compendium of uniformly processed human gene expression and splicing quantitative trait loci. Nat Genet53, 1290–1299 (2021). https://doi.org/10.1038/s41588-021-00924-w